EDHEC PhD in Finance alumnus Marat Molyboga has just released a book on Quantitative Hedge Fund Investing
In this interview, Marat Molyboga, EDHEC PhD (2019) tells us how he came up with the idea of writing the book* “Your Essential Guide to Quantitative Hedge Fund Investing” co-authored with Larry E. Swedroe
*published by Chapman & Hall (July 2023)
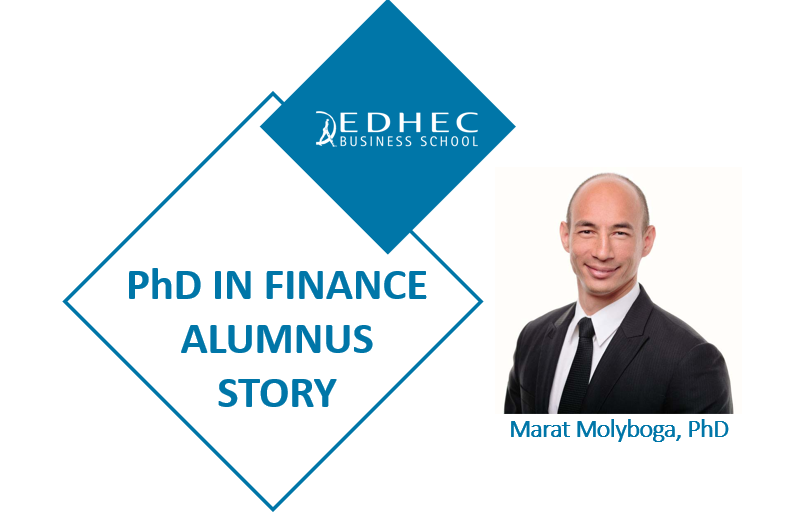
Dr Marat Molyboga is Chief Risk Officer and Director of Research at Efficient Capital Management. He is a seasoned investment professional with substantial experience in the area of quantitative research, systematic futures trading, risk premia, risk management, and portfolio construction. His research is published in academic and industry journals such as the Journal of Portfolio Management, Journal of Futures Markets, Journal of Financial Data Science, and Journal of Alternative Investments.
What inspired you to write this book?
I wanted to write this book for two reasons. First, I have been fortunate to work and collaborate with brilliant investment professionals and academics who passionately approach the topic of hedge fund investing with both scientific curiosity and rigor. They have shown me that quantitative hedge fund investing is full of widely accepted myths that must be debunked, and fascinating and challenging problems that can be successfully solved to benefit investors. Therefore, I wanted to write this book to honor those people and give back to others.
The second reason is a little selfish. I am very passionate about learning, and I was excited about this incredible opportunity to attain a deeper level of my own understanding of some of the most challenging and exciting topics of manager selection and portfolio construction. As the Roman philosopher Seneca said: “While we teach, we learn.”
I approached Larry to help me write this book because he has dedicated his life to educating investors about evidence-based investing.
In all of his books, Larry has been successful at distilling hundreds of heavy academic papers into pragmatic toolboxes of research-based investment ideas and decisions.
It seems that the EDHEC community (faculty and PhD graduates) was involved in this adventure, could you please explain how?
I have been very fortunate to receive a lot of support from the EDHEC community while at school and working on my book. Although I had more than ten years of experience in quantitative hedge fund investing before I joined the Ph.D. program at EDHEC in 2015, the Empirical Finance class taught by Rene Garcia covered several fascinating techniques that I ended up including in my book. I was fortunate to learn a lot from Abraham Lioui and my advisor Laurent Calvet who patiently guided my studies and shared relevant research with me. I am particularly grateful to Raman Uppal, who has been very kind and generous with his time. In my book, I discuss several Raman’s research papers that every quant should read and thoroughly understand because of their deep insights and practical implications. Raman was quick to answer my questions about some of the challenging aspects of his papers and also recommended other papers that were particularly helpful. After reading a draft of my book, Raman wrote a nice endorsement that I included in the book.
I also received a lot of help from my Ph.D. classmates. Mark Refermat and Hong Sherwin encouraged me to write the book when I had doubts. Jasmine Yu, who is a world-class expert in manager selection, shared many practical suggestions with me. Nikolai Semtchouk carefully read the manuscript and recommended many changes. David Mascio gave me several ideas for factor selection based on his research with Frank Fabozzi. Finally, Frank Fabozzi was very kind to write a nice foreword for my book. I don’t think I would’ve been able to finish my book without all the help I received from the EDHEC community.
Who should read this book?
We wrote this book to help all investors better understand and manage hedge fund portfolios. Although many of the topics covered in our book are highly technical, we wanted to make it accessible to any hedge fund investor who is interested in evidence-based investing. To accomplish this objective:
• We were intentional about choosing simplicity over technical precision.
• We italicized the first use of technical financial, statistical, or machine learning terms and provided their definitions in a glossary.
• We summarized key takeaways at the end of each chapter.
• We included a list of all referenced academic papers at the end of each chapter.
We also wanted our book to be useful for the quants and graduate students who are interested in the highly technical aspects of investing that are required when implementing and testing sophisticated portfolio management approaches. Thus, we included four appendices that provide in-depth technical descriptions and implementation steps for most of the approaches covered in the book.
What does this book cover?
Hedge fund investing is often misunderstood. In our book, we debunk several myths: hedge fund investing and selection of top performers are easy; hedge funds hedge; active and socially responsible hedge funds outperform; and investors benefit from hedge funds identifying undervalued stocks.
Empirical hedge fund research is performed by academics and practitioners. While each perspective is valuable, they are also incomplete—making it vital to appreciate and blend both views in order to build robust hedge fund portfolios. We show that hedge fund researchers can use one or several databases. However, the quality of the databases varies significantly and empirical data should be carefully evaluated and adjusted for biases. In addition, investors should either allocate via managed accounts or carefully account for rebalancing frictions in their investment process.
After a deep-dive into the empirical data, we turn to the fascinating and challenging topic of hedge fund selection. We demonstrate that fund selection should be customized to the specific objectives and constraints of each unique investor. We also show the importance of identifying hedge funds with a positive marginal impact on the existing portfolios. We then discuss how machine learning offers several promising approaches that can help with selecting a subset of factors that are relevant for a given hedge fund. However, quantitative performance evaluation is challenging because of the role of serendipity. Therefore, a hedge fund investor should also consider qualitative factors.
We also highlight the need to perform specialized operational due diligence of digital asset funds.
When we discuss portfolio construction, we also show the importance of customizing portfolios. For example, pension plan investors can benefit from explicitly evaluating the impact of candidate investments on their funding ratios. Although most portfolio techniques perform poorly out-of-sample, hedge fund investors can improve performance by diversifying risk across strategies and targeting volatility.
Investors may also consider top-down portfolio approaches or recent cutting-edge techniques covered in the book. However, it is important to not be over-reliant on recent historical data. When the market environment changes, historical periods with a similar environment, rather than the most recent period, are more relevant for forward-looking portfolio decisions.
Although we have learned a lot from the challenging process of distilling hundreds of heavy academic papers into pragmatic toolboxes of research-based investment ideas and decisions, we particularly enjoyed gaining insights from the thought leaders in modern finance who generously contributed to our book. The last few chapters of the book are unusual for quantitative manuscripts. They share experiences and suggestions of accomplished hedge fund managers and investors who kept on searching for answers in their respective areas despite obstacles and setbacks. Their stories also have a common thread of diversity, inclusion, and fulfillment from helping others. We hope that their stories will inspire young people to pursue their dreams, even if the odds may be stacked against them.